PhD dissertation: Robust state estimation methods for robotics applications[pdf]

Analysis of Scale-Variant Robust Kernel Optimization for Non-linear Least Squares Problems[paper]
In this article, we present a method for increasing adaptivity of an existing robust estimation algorithm by learning two parameters to better fit the residual distribution. The analyzed method uses these two parameters to calculate weights for Iterative Re-weighted Least Squares. This adaptive nature of the weights can be helpful in situations where the noise level varies in the measurements. We test our algorithm first on the point cloud registration problem with synthetic data sets and LiDAR odometry with open source real-world data sets. We show that the existing approach needs an additional manual tuning of a residual scale parameter which our method directly learns from data and has similar or better performance. We further present the idea of decoupling scale and shape parameters to improve performance of the algorithm. We give detailed analysis of our algorithm along with its comparison with similar well-known algorithms from literature to show the benefits of the proposed approach.
Authors: Shounak Das, Jason Gross
In this work, we demonstrate the importance of zero velocity information for GNSS-based navigation. The effectiveness of using the zero velocity information with ZUPT for inertial navigation applications has been shown in the literature. Here we leverage this information and add it as a position constraint in a GNSS factor graph. We also compare its performance to a GNSS-INS coupled factor graph. We tested our ZUPT aided factor graph method on three datasets and compared it with the GNSS-only factor graph.
Authors: Cagri Kilic, Shounak Das, Eduardo Gutierrez, Ryan Watson, and Jason Gross
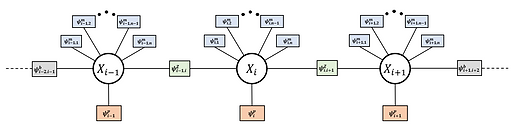
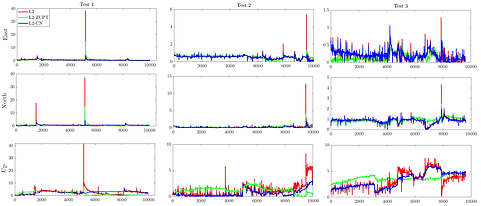


A Comparison of Robust Kalman Filters for Improving Wheel-Inertial Odometry in Planetary Rovers [paper][code]
This paper compares the performance of adaptive and robust Kalman filter algorithms in improving wheel-inertial odometry on low featured rough terrain. Approaches include classical adaptive and robust methods as well as variational methods, which are evaluated experimentally on a wheeled rover in terrain similar to what would be encountered in planetary exploration. Variational filters show improved solution accuracy compared to the classical adaptive filters and are able to handle erroneous wheel odometry measurements and keep good localization for longer distances without significant drift. We also show how varying the parameters affects localization performance.
Authors: Shounak Das, Cagri Kilic, Ryan Watson, and Jason Gross